Collaboration Capacity: A Framework for Measuring Data-Intensive Biomedical Research (2020-2022)
The goal of this proposed project is to develop a collaboration capacity framework and evaluate the collaboration capacity of science teams at macro-, meso-, and micro-levels through using GenBank metadata and other related data sources. The framework defines the Scientific & Technical (S&T) human capital, cyberinfrastructure, and science policy as the enablers of collaboration capacity, the impact of which on collaboration capacity can be measured by data production and data-to-knowledge metrics such as team size and ratio of data to publications. GenBank metadata as the primary data source for this project offers a longitudinal coverage (1984-2018) and full research lifecycle traces from data production to publication to patent application, creating an unprecedented opportunity to study the biomedical research enterprise.
This project will design and create datasets from GenBank metadata to generate analysis-ready data, which will be combined with statistics from NSF and NIH. The datasets will be used to develop computational models and test hypotheses that examine the correlation between collaboration capacity, team size, and connectedness of nodes, as well as the properties of disruptive nodes and their impact on productivity and innovation. In addition to statistics from NSF and NIH, the project will also combine events in science policy (e.g., mandates on data sharing), public health (e.g., outbreaks and prevalent chronic diseases), and funding to triangulate with the datasets and analyze collaboration capacity and policy implications. The data source and theoretical approach compensate for the limitations of publication-centric data sources used in past research on collaboration networks. The fact that the primary data source comes from basic biomedical research situates this study at the cutting-edge and allows us to gain more holistic insights into the impact of federal investment and policy on collaboration capacity. Our future research will use this longitudinal, rich data collection to continue deeper mining of collaboration in data production and data-to-knowledge lifecycle, particularly in relation to specific genes, diseases, and treatments that are key aspects in basic and clinical biomedical research.
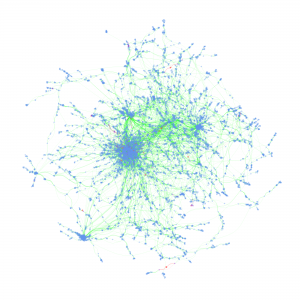
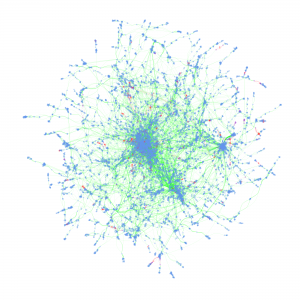
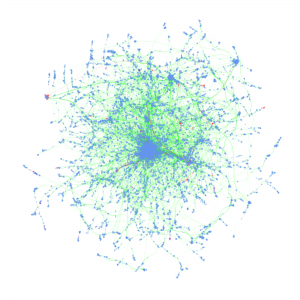
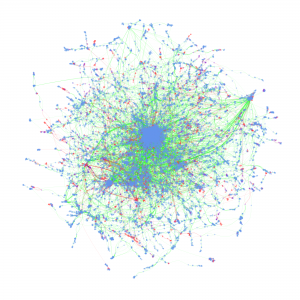
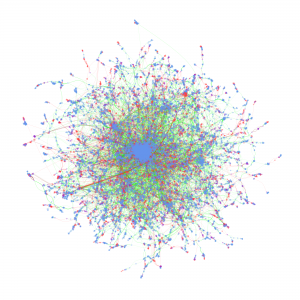
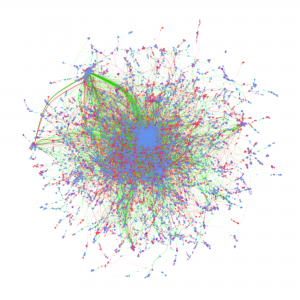
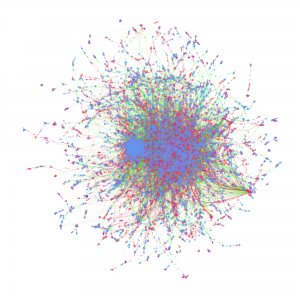
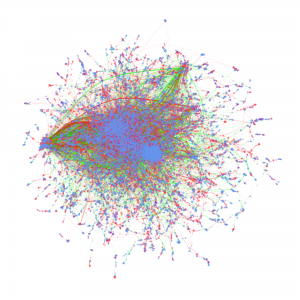
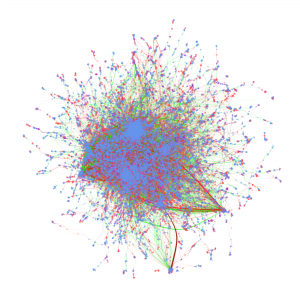
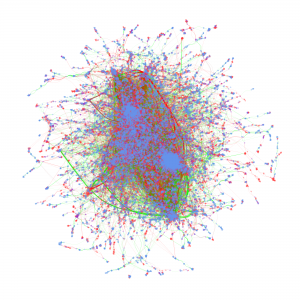
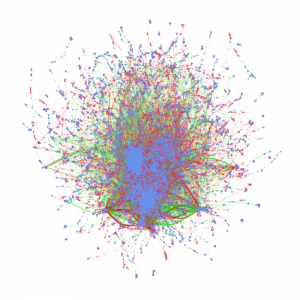
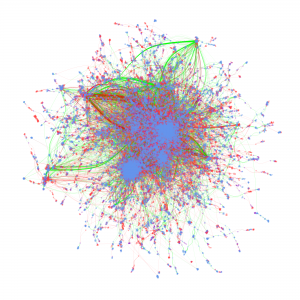
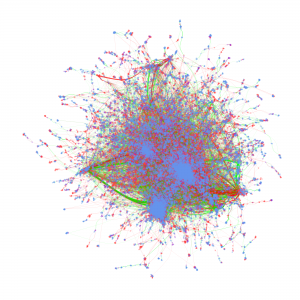
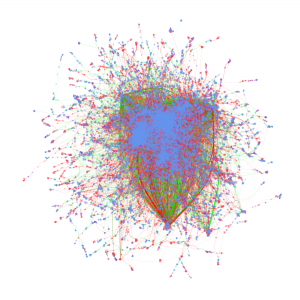
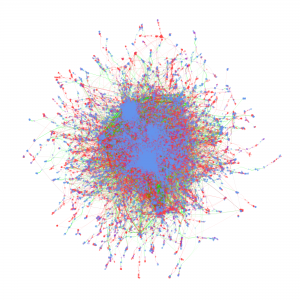
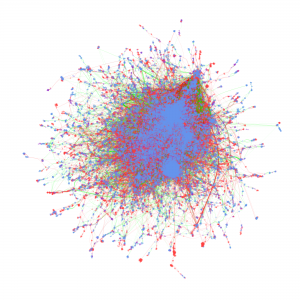
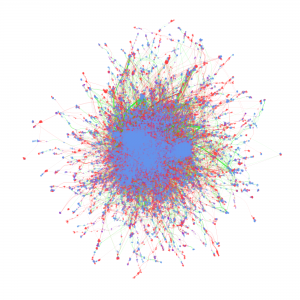
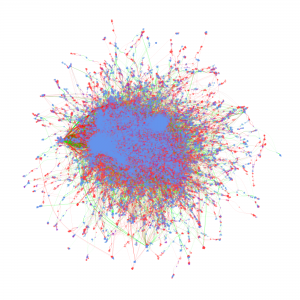
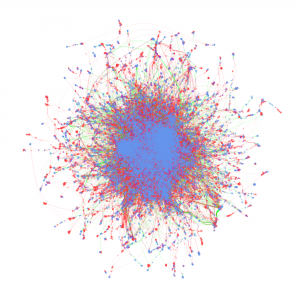
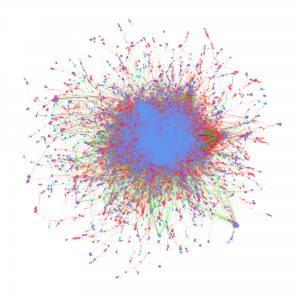
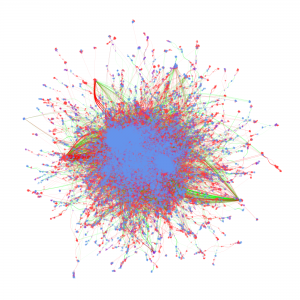
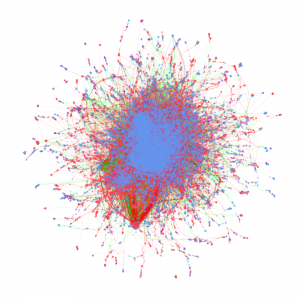
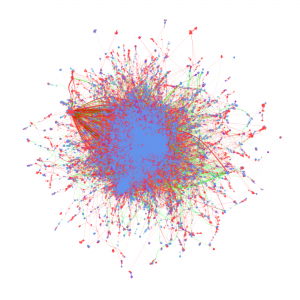
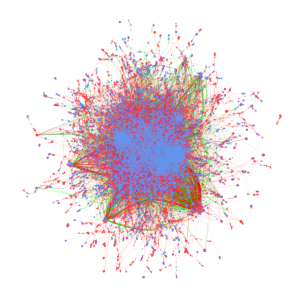
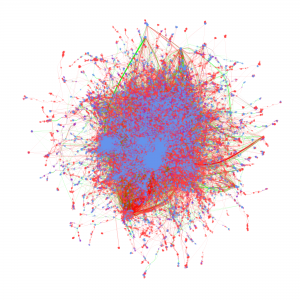
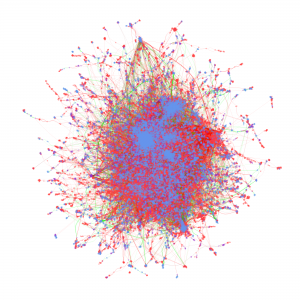
Cyberinfrastructure-Enabled Collaboration Networks (2016-2019)
Cyberinfrastructure enables collaborative research and significantly impacts scientific capacity and knowledge diffusion. In response to the growing need for quantitatively evaluating outcomes and impact of federal investment on research, this project deploys new data, tools, metrics, and methods for assessing the impact of cyberinfrastructures and the data services built on them. This research helps researchers and policy makers understand how cyberinfrastructure affects collaboration dynamics and network structures of researchers. Datasets organized by longitudinal, thematic, topical, geographical, institutional, and author dimensions provided, which researchers, policy makers, and students can access and use to explore data-intensive science of science and innovation policy related research.
Metadata from GenBank, patent data from U.S. Patent and Trademark Office and funding data from NIH ExPORT are analyzed with descriptive statistics and models from Complex Network Analysis. The project not only examines the topological properties of the data submission and publication networks, but also the temporal ordering of collaborative relationships and the overlap of the sequence submission and publication networks. Through slicing, plotting, and visualizing data, appropriate sampling strategies and algorithms are developed to more deeply explore collaboration networks, both structurally and temporally. Algorithms used in community detection, machine learning, and visualization serve as primary computational methods in this research. Data products to be shared with research communities include 1) discovery lifecycle datasets containing sequence submissions, publications, and patents as well as the links between them and 2) funding factor datasets containing links between U.S. federal funding data and the discovery lifecycle datasets.
FUNDED BY: NIH/NIGMS
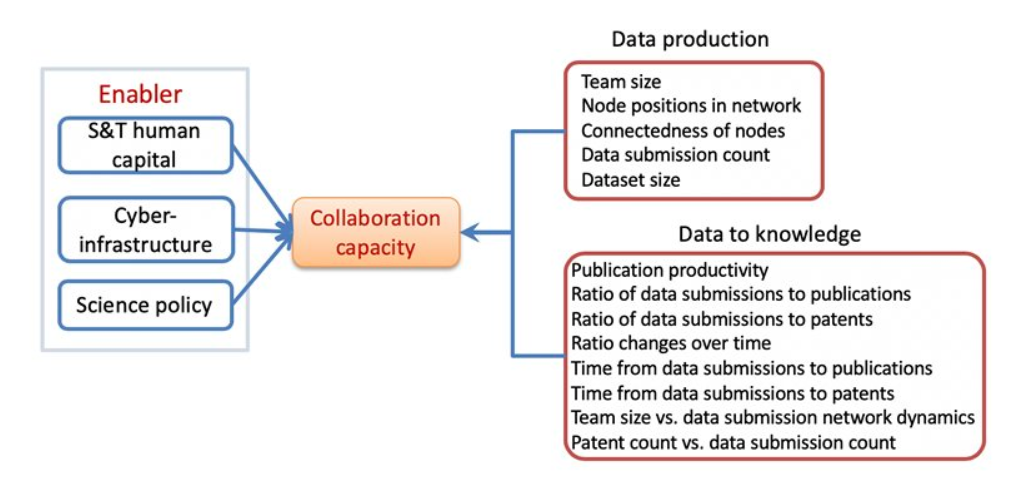
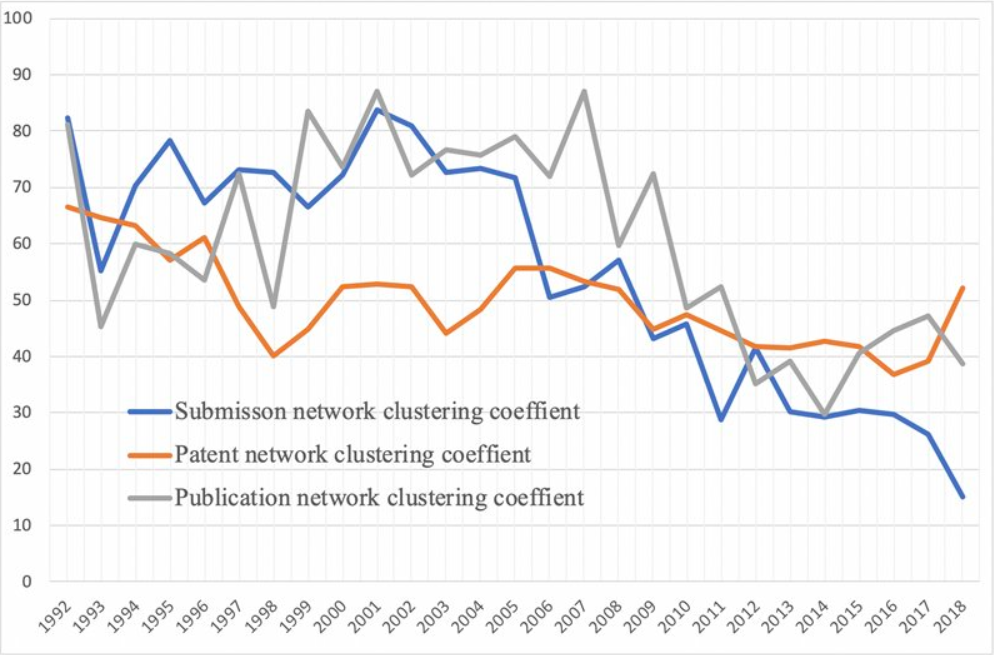
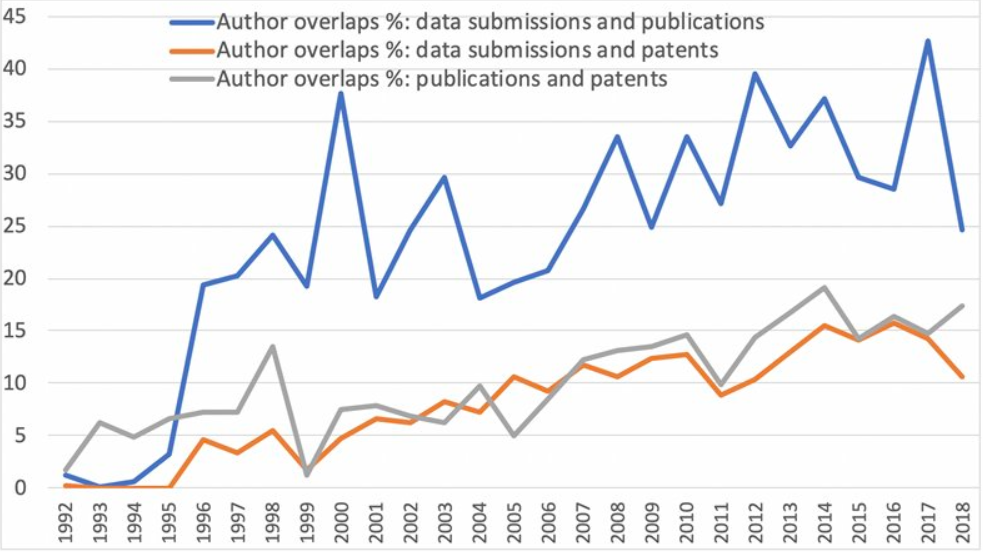
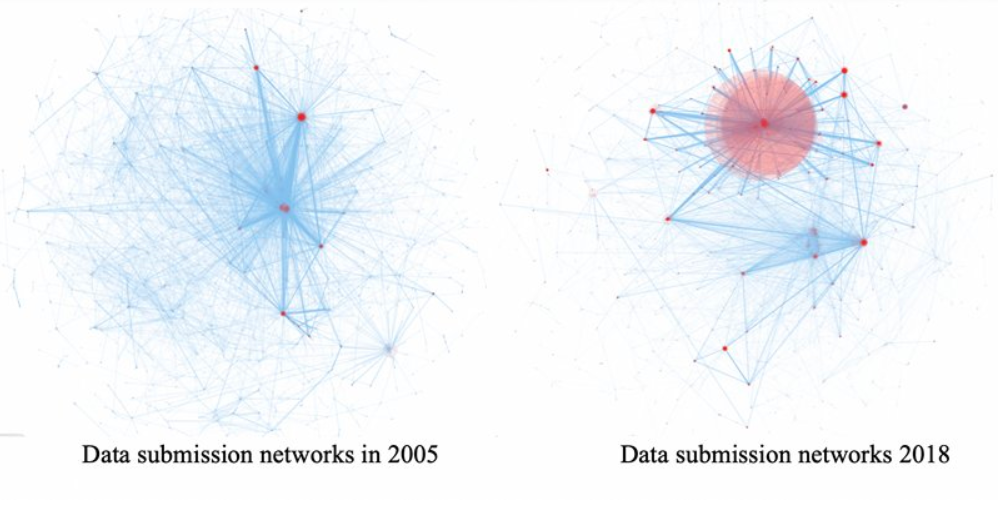
- Bratt, S. E., Qin, J., Hemsley, J., Amol , S., & Devitt, W. (2021). Data-Publication Collaboration Networks in HIV Research. Long paper submitted to ASIST Annual Conference
VIEW PUBLICATION >>>
- Hemsley, J., J. Qin, & S. Bratt. (2020). Data to knowledge in action: A longitudinal analysis of GenBank metadata. In: Proc. Assoc. Info. Sci. Tech.https://doi-org.libezproxy2.syr.edu/10.1002/pra2.253
VIEW PUBLICATION >>>
- Bratt, S.E., Hemsley, J., & Qin, J. (2019). A closer look at the data co-authorship: Trends in team size in “big science”. In: The 17th International Society of Scientometrics and Informetrics Conference, Rome, Italy, September 2-5, 2019. https://www.issi-society.org/proceedings/issi_2019/ISSI%202019%20-%20Proceedings%20VOLUME%20I.pdf
VIEW PUBLICATION >>>
- Dobreski, B., M. Resnick, & J. Qin. (2019). Side by side: The use of multiple subject languages in capturing shifting contexts around historical collections. In: International Society for Knowledge Organization: Chapter for Canada and United States, June 13-14, Philadelphia, PA, June 13-14, 2019 (NASKO 2019). https://journals.lib.washington.edu/index.php/nasko/article/view/15615
VIEW PUBLICATION >>>
- Qin, J., J. Hemsley, & S. Bratt. (2018). Collaboration capacity: Measuring the impact of cyberinfrastructure-enabled collaboration networks. Science of Team Science (SCITS) 2018 Conference, Galveston, Texas, May 21-24, 2018 https://experts.syr.edu/en/publications/collaboration-capacity-measuring-the-impact-of-cyberinfrastructur
VIEW PUBLICATION >>>
- Bratt, S., Hemsley, J., Qin, J., & Costa, M. (2017). Big data, big metadata and quantitative study of science: A workflow model for big scientometrics. Proceedings of the Association for Information Science and Technology, 54(1), 36–45. https://doi.org/10.1002/pra2.2017.14505401005
VIEW PUBLICATION >>>
- Bratt, S.E., Costa, M. Hemsley, J., & Qin, J. (2016). Validating science’s power players: Scientometric mixed methods for data verification in indentifying influential scientists in a genetics collaboration community. iConference, Philidelphia, PA, March 2016.
VIEW PUBLICATION >>>
- Costa, M. R., Qin, J., & Bratt, S. (2016). Emergence of collaboration networks around large scale data repositories: A study of the genomics community using GenBank. Scientometrics, 108(1): 21-40. DOI: 10.1007/s11192-016-1954-x
VIEW PUBLICATION >>>
- Costa, M. R. (2016). The interdependence of scientists in the era of team science: An exploratory study using temporal network analysis. Dissertations – ALL. 425. https://surface.syr.edu/etd/425
VIEW PUBLICATION >>>
- Qin, J., Costa, M., & Wang, J. (2015). Methodological and technical challenges in big scientometric data analytics. iConference 2015, Newport Beach, CA, March 24-27, 2015. http://hdl.handle.net/2142/73756
VIEW PUBLICATION >>>
- Qin, Jian; Hemsley, Jeff; Bratt, Sarah, 2021, “GenBank data submission network yearly data frame files”, https://doi.org/10.7910/DVN/4QUAXY, Harvard Dataverse, V1
ACCESS DATA >>>
- Qin, Jian; Hemsley, Jeff; Bratt, Sarah, 2021, “GenBank publication network yearly data frame files”, https://doi.org/10.7910/DVN/YGWKLA, Harvard Dataverse, V1
ACCESS DATA >>>
- Qin, Jian; Hemsley, Jeff; Bratt, Sarah, 2021, “GenBank network assortative mixing R data frames”, https://doi.org/10.7910/DVN/ZRVK1L, Harvard Dataverse, V1
ACCESS DATA >>>